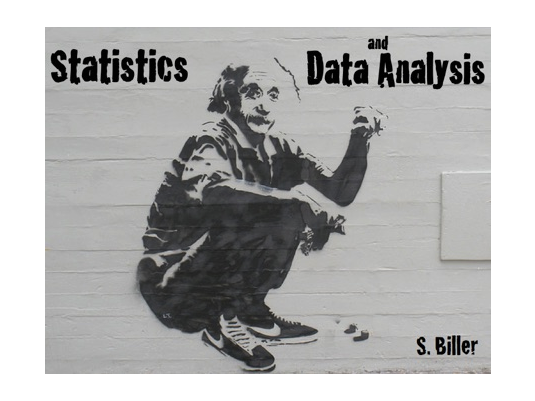
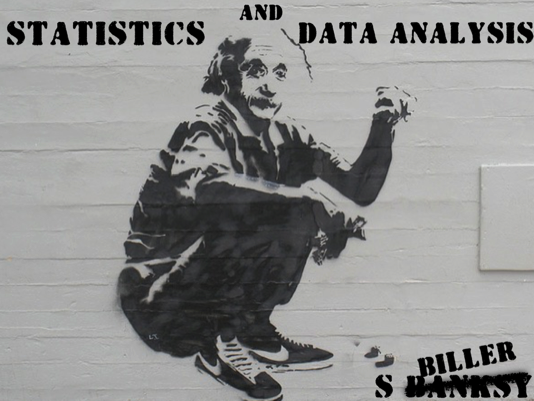
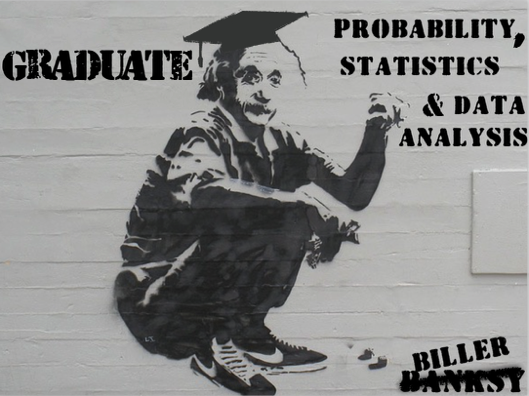
OVERVIEW
Lecture 1: Probability traps; Binomial & Poisson Distributons; Expectation and Variance; Estimators; Gaussian Distribution
Lecture 2: Central Limit Theorem; Properties of Normal Distributions; Look elsewhere effect; Regression to the Mean; Correlations
Lecture 3: Uncertainties & Error Propagation; Testing Models: chi-squared; “Scientific Method”
Lecture 4: Student’s t; Correlation test; Non-parametric tests: rank and K-S; What is ‘Normal?’; Robust parameter estimation
Lecture 5: p-values; Combined p-values as a Statistic; Maximum Likelihood; Neyman-Pearson Lemma; Wilks' Theorem; Joint analysis of multiple data sets; Extended Likelihood; Nuisance parameters and profile likelihood
Lecture 6: Asimov data sets; Propagating PDF uncertainties; Bayes’ Theorem
Lecture 7: Confidence and Credibility intervals: Asking the right question; Wilks (again); Neyman construction; “Standard” and “Feldman-Cousins,” Interpretation issues; Bayesian intervals and Priors
Lecture 8: Loose Ends: Real ensembles; Constant priors; Robust data comparisons; Data Presentation: ‘Binsmanship’ and Dodgy Error Bars; More Things to Avoid; Ways to Display Uncertainties; Visualising Multi-Dimensional Data; Boxes, Whiskers and Violins
Lecture 9: Blind Analysis; Bifurcated side-band analysis, Data “Correction”; Statistical Optimisation; Redundancy
Lecture 10: Monte Carlo methods: Distribution sampling; Markov chains; MC Integration; Smart sampling, Weighted sampling
Lecture 11: Optimisaton Methods: Grid Search; Golden Ratio; Powell's Method, Gradient Descent; Determination of posterior densities via Markov Chain Monte Carlos
Lecture 12: Fisher Linear Discriminant, Decision trees & boosted decision trees
"Data Analysis in High Energy Physics: A Practical Guide to Statistical Methods",
Behnke et al. (2013)“
Statistical Data Analysis”, G. Cowan (1998)
"Numerical Recipes", W. Press, S. Teukolsky, W. Vettering & B. Flannery (2007)
“A Guide to the Use of Statistical Methods in the Physical Sciences”, R. J. Barlow (2008)
“Data Analysis: A Bayesian Tutorial”
by D. Sivia (1996)
“Measurements and their Uncertainties: A practical guide to modern error analysis” by I. Hughes and T. Hase (2010)
"A Student's Guide to Bayesian Statistics" by Ben Lambert (2018)
“Probability Theory: An Introductory Course” by Ya. G. Sinai (1992)
SUGGESTED BOOKS